Data quality is a crucial factor for the success of any data-driven company. But how does one accurately assess an organization’s data maturity level to be able to better represent the importance of fixing gaps and opportunities for improvement to executives within a company?
In one of our previous articles, we already discussed the 6 core dimensions that can be used to measure your data quality. In today’s article, you will learn more about how to assess your organization’s data situation to know where to go from here. For it, you will learn about the data quality maturity model, a framework that helps businesses assess and evaluate how well their company is leveraging data quality capabilities and performance based on six other dimensions: data governance, data architecture, data acquisition, data validation, data transformation, and data usage.
Table of Contents
Navigating the 4 Phases of Data Maturity
Data maturity encompasses four different levels that aim to benchmark your current state, identify your strengths and weaknesses, and prioritize actions for improvement.
However, it is important to consider that data quality maturity models not only focus on how accurate data is. Instead, as DJ Patil and Hilary Mason emphasize in their book Data-Driven: Creating a Data Culture, it evaluates how companies use data as an asset that is embedded into the company's culture DNA to generate value and outcomes.
In this sense, each phase is evaluated and assessed by a combination of strategic, operational, and cultural data-driven practices. Let’s have a closer look at each of them.
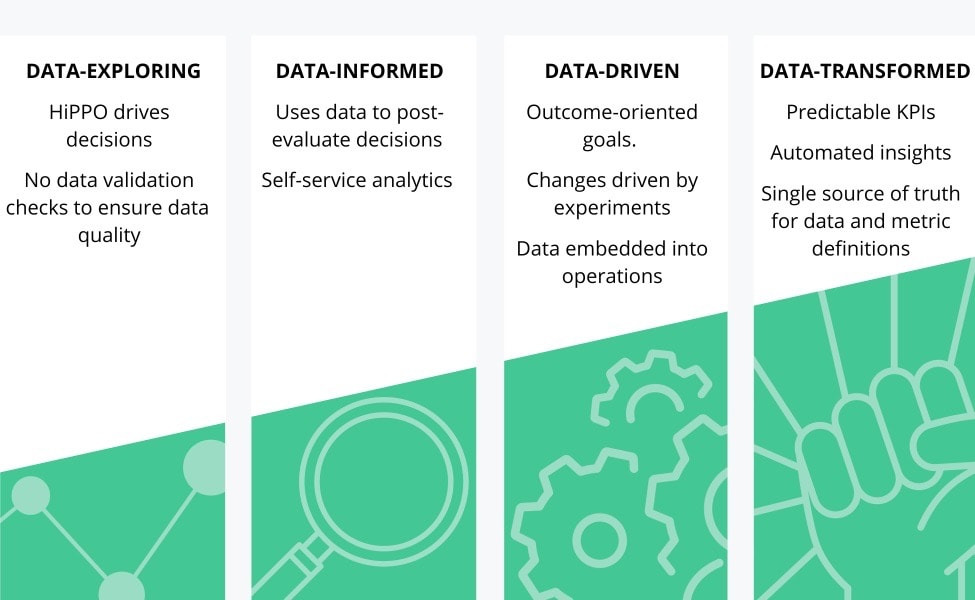
Phase 1: Data-exploring
This is the starting point that many companies follow as they mature digitally, and basically revolves around exploring what data you have.
Maybe you’ve just started collecting and reviewing some simple KPIs, but you’re far from unlocking all the potential that data can offer for strategic-decision making. Indeed, at this level of data maturity, decisions are still often being made with little to no data, and what’s worse, without any data checks to validate the quality of the data that is being collected.
A situation that makes it very difficult when it comes to defending your next initiatives, which are often underestimated by the ”HiPPO effect” to impose the gut-based guesses of the Highest Paid Person’s Opinion in the Office.
Phase 2: Data-informed
If you’re in this phase, congratulations; you’re not alone in this anymore. Other members in your company and, most importantly, your leaders, are beginning to see the value in investing in analytics tools and growing your company’s data stack to leverage the potential of your data.
Things like establishing best practices for roadmap planning, adding success metrics to each brief or campaign, basic analytics training, and access to self-serve tools to answer day-to-day questions in real-time have finally become a standard.
Phase 3: Data-driven
This is when data access has been more democratized and embedded into the company's culture. Data informs strategic and operational practices, driving business KPIs and optimizing digital experiences. Decisions are rooted in past learnings, and roadmap planning aligns with business impacts.
Phase 4: Data-transformed
Data is now part of your organization’s DNA. Every team and process is totally focused on data, and there’s a culture of sharing it throughout your organization.
Silos between teams and functions are not blurred anymore, and you have a tracking plan that serves as a single source of truth for all teams and squads and ensures all your data points are accurately collected, responsibly managed, and integrated efficiently across teams and platforms.
How to assess your data quality maturity level?
Determining where you are in your data maturity journey is a key step to figuring out what you have to do next.
To help you understand and establish your starting point in a data strategy that will lead you to streamline your organization’s data capabilities, the following questions aim to guide you in the process of evaluating the maturity level of your data quality processes.
- Does your company have digital analytics and obtain KPIs from it?
- The above, and you use digital analytics to manage marketing campaigns and understand your customers' behavior?
- The above, and you have basic data quality processes?
- The above, and you implement data lakes and use other data sources to enrich reporting?
- The above, and you have advanced data quality tools and processes?
- The above, and you have processes and people responsible for data governance?
- The above, and you have implemented CDPs (Customer Data Platforms) to personalize user experiences?
- The above, and you develop your own digital products based on data?
Data quality maturity models: A comparison
There are several models of data maturity and analytics in literature and industry. Some notable models include Gartner's Analytics Maturity Model and DAMA's (Data Management Association) Data Maturity Model. Let’s compare them:
Gartner's Analytics Maturity Model
Gartner proposes a maturity model that helps data and analytics leaders identify the level of development their initiatives must achieve to support business objectives, providing a roadmap for improvements.
Gartner's model consists of four phases: Descriptive Analytics, Diagnostic Analytics, Predictive Analytics, and finally, Prescriptive Analytics.
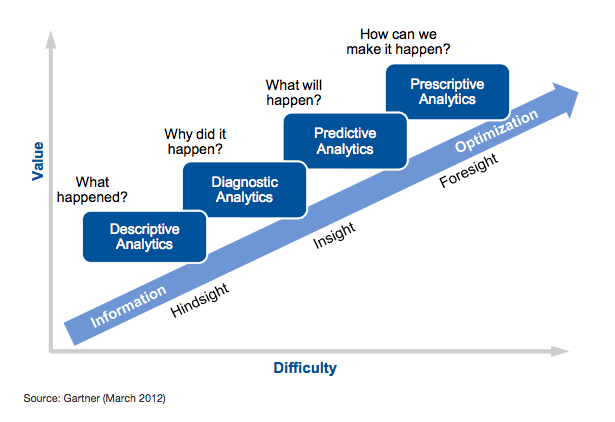
DAMA's Data Maturity Model
DAMA's Data Maturity Model employs an approach based on the Capability Maturity Model (CMM) originally developed by Carnegie Mellon University and is applied to assess and improve organizations' data management processes and procedures.
The maturity levels in this model are:
Immature
Best practice activities are not performed by the organization, and recommended tools are either unavailable or not used.
Repeatable
Some parts of the organization use recommended tools and processes, while others do not.
Managed
The organization has a documented standard for consistently and effectively performing assessed activities using applicable tools.
Monitored
The process in question is established, tracked, and monitored. Recommended tools are in place and consistently used throughout the organization.
Continuous Improvement: The activity is continually re-evaluated, improved, tracked, and integrated into the process.
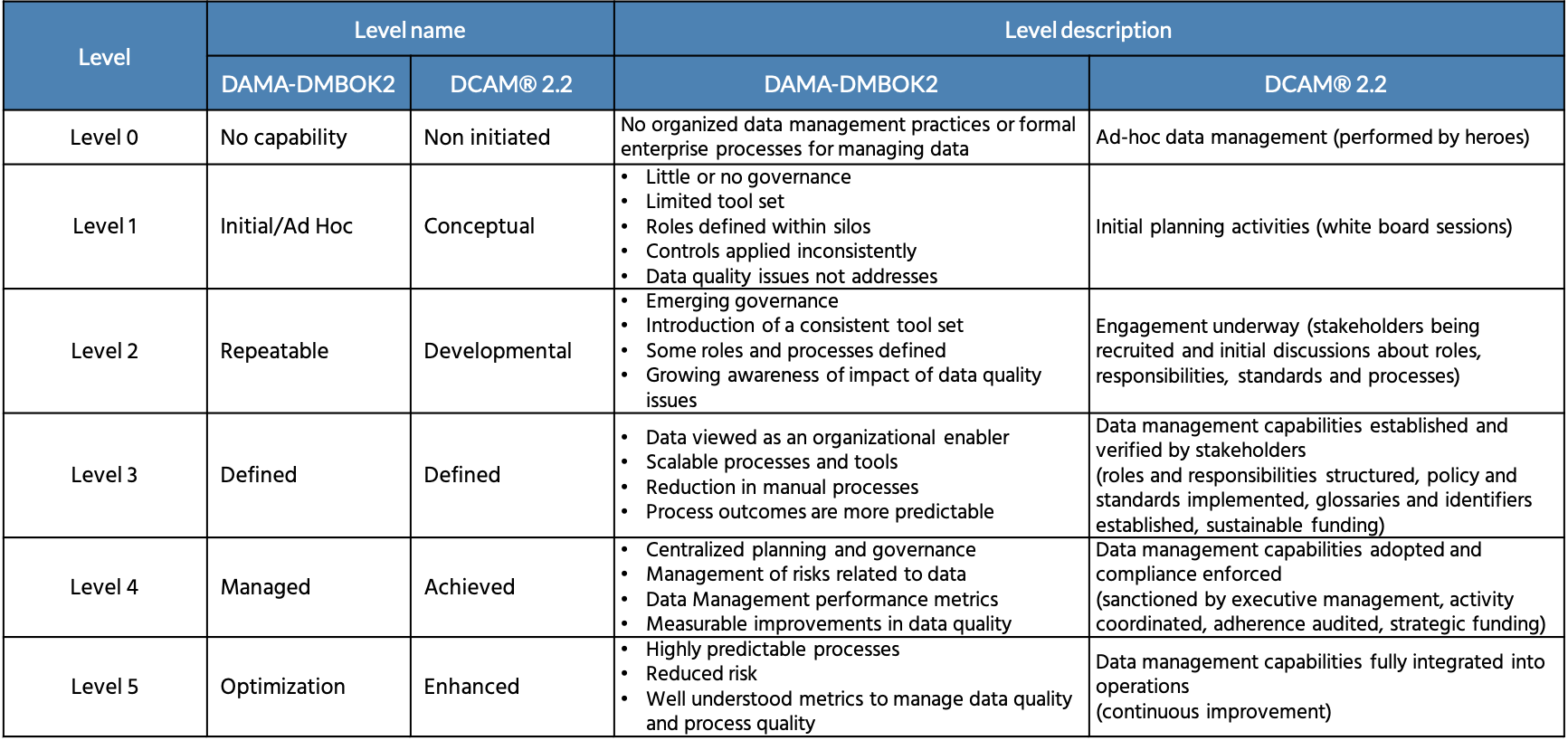
Conclusion
All in all, Data maturity models provide a structure and a frame of reference that could be very useful to contextualize the different levels of data usage within any company that wants to leverage their data processes and what steps can be taken to advance toward greater data usage maturity.
Trackingplan is a fully automated data QA and observability solution for your digital analytics created to ensure your data never breaks and always arrives to your specifications by automatically documenting all the data that your apps and websites are sending to third-party integrations like Google Analytics, Segment, or MixPanel.
Get started for free to experience the benefits of Trackingplan first-hand or ask for a demo.